The Impact of the Diffusion of Algorithms on Prices in Online Marketplaces: Efficiency or Collusion?
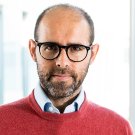
Francesco Decarolis
Principal Investigator
Team members: Christoph Carnell, Thomas Le Barbanchon
MIUR PRIN 2017
February 2020 - February 2024
Pricing decisions are increasingly in the “hands” of artificial algorithms. While these pricing algorithms have been used for a long time in specific markets like airline ticketing, only more recently their usage has expanded to other sectors, such as financial markets, hotels, insurance and essentially all online marketplaces organized as auctions or other biddable markets. This process has brought about substantial benefits in terms of efficiency of how prices can rapidly adjust to changes in demand and supply conditions, but has also created new challenges, especially with regard to the potential for tacit collusion. The current debate on algorithms and collusion has identified two main channels through which algorithmic pricing might enhance collusive behavior (see OECD, 2017). First, thanks to high price transparency and high-frequency trading, algorithms increase the ability to react fast and aggressively, thus making collusive strategies more stable in virtually any market structure. Second, by using automated mechanisms to implement common policies or optimize joint profits with deep learning techniques, algorithms can lead to the same outcomes of traditional “hard core” cartels through tacit collusion. This research projects aims at contributing to the understanding of how these two channels operate. It is articulated into two main components. One component focuses on online advertisement, the delivery of marketing messages via the Internet, analyzing how the auction systems where advertisement is sold are affected by the 'algorithmic bidding' practices of specialized intermediaries (Digital Marketing Agencies) to which advertisers increasingly delegate bidding. This component studies, both theoretically and empirically, the effects of agency bidding on the most commonly used auction formats (Google's GSP and Facebook's VCG auctions) focusing on the risks posed by joint profit maximization (i.e., tacit collusion) that occurs when competing advertisers bid through the same agency. The second component looks more in depth at how the most commonly used pricing algorithms (both “adaptive” and “learning” algorithms) can learn to collude. It studies, from both a theoretical and an experimental point of view, how pricing algorithms may coordinate on high prices, if collusion among algorithms is easier to sustain than among humans and what are the ensuing implications for competition policy. The first component builds on previous theoretical work by the PI (Decarolis et al., 2017) where a first set of theoretical results on agency bidding where established. There, we found that the agency's equilibrium bids are akin to implementing a certain form of collusion (even if none of its client explicitly attempt it), and that in this situation the VCG auction outperforms the GSP auction both in terms of revenues and efficiency. In the present work, the theoretical analysis will complement and expand these results through a more general characterisation of agency bidding involving the coalition formation stage and the simultaneous presence of multiple competing agencies. The empirical analysis, instead, will be based on a new dataset obtained by combining data on a large set of paid search auctions with information on the agencies to whom the advertisers in these auctions are affiliated. The first part of our empirical analysis will exploit the variation over time and across keywords in the presence of shared DMAs across advertisers to study through a reduced-form analysis whether the presence of shared DMAs causes the average cost-per-click to decline. A second part of the analysis will estimate a structural model of agency bidding in the GSP in order to obtain counterfactual estimates of the revenues and efficiency effects of collusive bidding. The second component explores a different but closely connected question: how do in practice pricing algorithms bolster collusion? Building on preliminary work by Calvano et al., (2018), this study looks a the functioning of pricing software powered by Machine Learning algorithms (ML). Their massive growth is due on the one hand to the entry in the supply chain of pricing service providers which offer affordable personalized solutions and on the other hand to the availability of “off the shelf” ML products and services at low prices by tech giants such a Google or Microsoft. This component is a first step at defining, identifying and studying the possibility and the juridical consequences of algorithmic collusion. We plan to address the following broad questions: can Autonomous Agents interacting in a marketplace “learn” to collude? Which classes of algorithms support supra-competitive prices? Which kind of strategies emerge and how do these relate to the collusive strategy profiles already identified in the literature? Finding that algorithms enhance collusion will then lead us to explore the legal implications of our analysis.
Other Research Unit:
- Università degli Studi di Bologna - Coordinator: Emilio Calvano
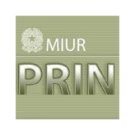
This project has been funded by Ministero dell'Istruzione, dell'Università e della Ricerca under the framework PRIN 2017 - Progetti di ricerca di Rilevante Interesse Nazionale.